Guaranteed AI and approximate AI
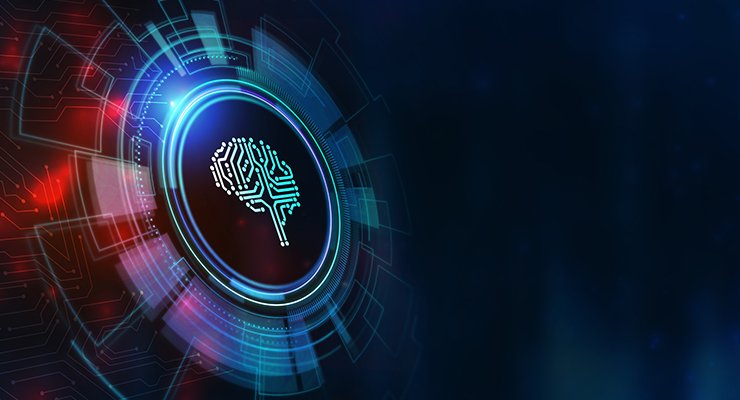
Sébastien Destercke is a CNRS research scientist and head of the Knowledge, Uncertainty, Data (CID) team at Heudiasyc, a joint UTC/CNRS research laboratory. He is also the titular holder of the Industrial Chair in Artificial Intelligence (AI) launched at the beginning of 2022.
In addition to UTC, this chair involves The Sorbonne Center for Artificial Intelligence (SCAI), renamed Sorbonne Cluster for Artificial Intelligence, the CNRS and Sopra Steria, founding sponsor of the UTC Foundation for Innovation. At UTC, this chair mobilizes two laboratories working on AI itself — Heudiasyc’s CID (Knowledge, Uncertainty, Data) team and LMAC (Applied Maths), whose work is partly at the heart of AI — and three others – UTC-Roberval, UTC-BMBI (Biomechanics and Bioengineering Laboratory) and UTC-Avenues — specialized in fields where AI applications are now playing a growing role. Heudiasyc is also involved in AI applications.
There isn’t just a single AI, but many AI models, each tailored to specific applications. “For all these models, there are two main underlying trends: guaranteed reasoning and approximate reasoning. Statistical methods, the most widely used at present, are part of approximate reasoning. When these models make predictions, they can only do so with statistical guarantees. Conversely, existing calculation methods in processors, as well as a certain number of verifiers in computers, or even assignments in Parcoursup , are part of guaranteed reasoning. In short, we need to be sure that a given input will always correspond to the same output”, he explains.
Take predictive AI systems. “The question is to know whether their predictive capacities are in line with what has been observed in the real world, for example, in the field of medical diagnosis. In this case, it’s essential to add a fair estimate of certainty to the prediction, so that the information provided is as useful as possible”, he adds.
So what about generative AI, the latest AI technology? “This technology needs huge quantities of data, which it will structure in neural networks. Generative AI will build, generate plausible outputs from the data they have. If I take ChatGPT, for example and give it a text to illustrate, it will create a new image from images and texts close to the one to be illustrated. This means that these methods have no requirement for veracity. If I ask him for a piece of information often found in his data, such as the year of Charlemagne’s birth, he’ll answer around the year 800. On the other hand, if I ask it to translate a text in a rare language of which it has seen few texts, it will generate a translation, but I doubt it will be accurate. A possible disadvantage of this AI is that its operation is not intelligible. It’s often referred to as a “black box”. Coupled with the fact that it does not seek to predict reality, it is therefore dangerous to use it in certain fields, such as medicine or transport, unless it is coupled with other systems that make up for its shortcomings. You have to think of it as an assistant, not as a decision-maker,” he stresses.
Finally, whether generative or predictive, another current problem with AI is that it can generate or reproduce damaging biases. This is what happened, for example, with Amazon’s HR [human resources] tool, which tended to favour male applicants because, at the outset, it was mainly men who applied, so they are more numerous in the database.
Among the tools developed at Heudiasyc? “Personally, my work focuses on models that can approach reality. Models that are robust and reliable, and that provide me with sufficient statistical guarantees. These two criteria are part of the broader issue of trusted AI. The first is a decisive aspect in many industrial applications, and particularly in AI systems. Their robustness is measured by their ability to adapt to changing deployment conditions and environments, without losing quality. The second is to quantify the uncertainty associated with the system’s predictions. The aim is for the system to be able to quantify its own confidence in its predictions. If the system gives a 90% accurate prediction, I’d like it to be accurate 90% of the time. If we take the autonomous, i.e., driverless car as an example, it’s obvious that trusted AI is essential,” asserts Sébastien Destercke.
What are the challenges for the next generation of AI? “One of them will undoubtedly be to be able to combine guaranteed AI with the extrapolation and interpretation capabilities of generative AI, in particular LLM (large language model) models”, he concludes.
MSD