Tools to prevent certain medical risks
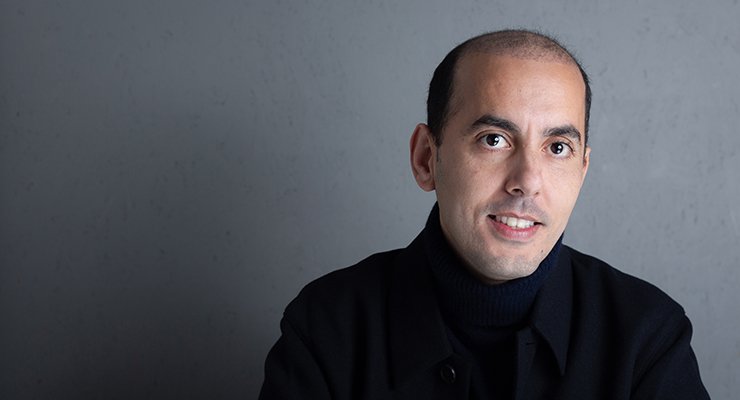
Imad Rida is a senior lecturer and research scientist at UTC-CNRS-BMBI in the C2Must team. He contributes to the development of AI models adapted to various research projects, notably the prevention of premature birth and muscle aging.
After completing a thesis on machine learning and more specifically on representation learning for classification, at INSA Rouen, Imad Rida remained there for two years as a contract teacher-research scientist (ATER). In 2019, he was recruited to become a lecturer at UTC. His accumulated experience in AI has enabled him to develop a number of tools adapted to specific problems. “This is the case for the prevention of premature childbirth with Dan Istrate and Catherine Marque, for example, or the assessment of aging of the musculoskeletal system with Sofiane Boudaoud. In these two areas, my aim has been to introduce new AI techniques based on data, often electrical signals, collected using electrodes called HD-sEMG. I use AI techniques to analyse the data collected, as the data collection is carried out by other players, such as maternity wards for pregnant women, for example”, explains Imad Rida.
Among the specific tools developed? “When there is little data, I use parsimonious representation learning techniques. This is known as dictionary representation learning. However, when there’s a lot of data available, deep learning techniques are used. We can, however, use deep learning in the first case, by using techniques known as “data augmentation”, by multiplying the interactions between the available data, or we can use generative AI. In my various projects, I’m mainly working on classification tools. Let’s take the case of muscle ageing, where we define age classes: 20- 30 years, 30–40 years, 40–50 years and so on. We know that the age of a muscle can differ from its actual age. We have a database with the muscular characteristics of different people of different ages and, when we recruit a new subject, the AI will assign him a class. This may be higher than the subject’s actual class, for example, because the data collected from the subject’s HD-sEMG shows that, for reasons of sedentariness or lack of physical exercise, the state of the subject’s muscle does not reflect his or her real age”, he explains.
AI tools are constantly evolving, and other techniques are bound to develop to meet specific uses. “AI is certainly a most welcome aid to decision-making and diagnosis. But vigilance is still required, particularly in terms of confidentiality and data protection”, concludes Imad Rida.
MSD