Preventing falls through deep generative learning
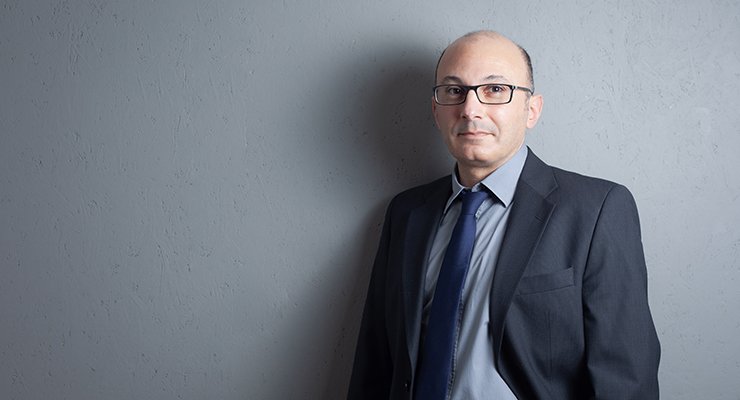
Karim El Kirat, a university professor, is co-head, with Sofiane Boudaoud, of the C2MUST team at UTC-CNRS-BMBI. He develops multi-scale models for fall prevention, using AI in particular.
It was with Tuan Dao, then a member of UTC-BMBI and an AI specialist, that Karim El Kirat began working on multi-scale models at the molecular level. Since then, Tuan Dao has joined the Ecole Centrale de Lille as a professor, but their collaboration has continued on multi-scale modelling of the whole body. “The biomechanics of the human body integrates different aspects. We are interested in the musculoskeletal system, namely the muscles, bones and tendons. The objective is to define the role of the different elements during a movement, for example. The bone is the foundation of the system, the tendons are springs of a sort, while the muscles are actuators. The question is: who controls all this and how does it work? When we do multi-scale modelling, we simultaneously analyse how the muscle will contract at different scales to produce movement on the human scale, and how nerve impulses participate in all this,” explains Karim El Kirat.
The two research scientists were particularly interested in the problem of falling. “When we’re small, we learn to walk by falling over, getting up and continuing to walk, or so we think, for life. However, sarcopenia, the infiltration of fat into the muscle that lowers muscle strength, can begin as early as the age of 40, leading to an increasing risk of falling with age, especially for sedentary subjects. Thus, in the modelling, it was decided to use a biomimetic AI that mimics the process of learning to walk. A model based on a specific AI was developed: generative deep learning. A biomechanical model of the whole body is created, integrating the mechanical properties of bone, muscle and tendon. The model is then asked to take a few steps and to fall. The model is rewarded if it achieves the objectives (falling, or not) and it is thus possible to analyse which parts of the body, which stages of walking are decisive if we want to avoid falling. The model is trained using the data available in our database but also data from the literature in order to ultimately propose preventive musculoskeletal strengthening routines,” he concludes.
MSD