AI tools differentiated by specific applications
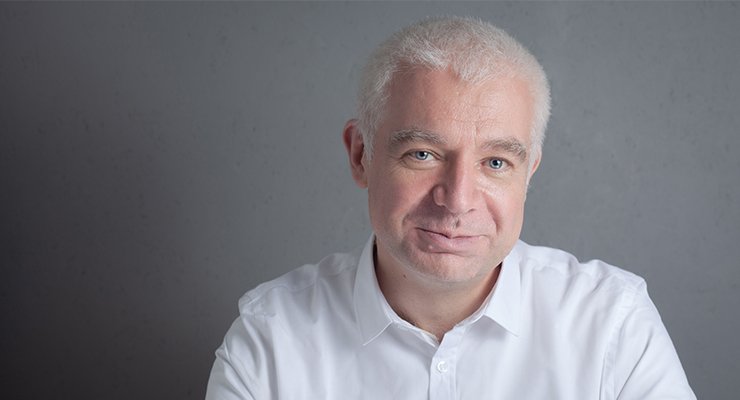
Florian De Vuyst is a university professor specializing in applied mathematics and a research scientist at the Biomechanics and Bioengineering Laboratory (UTC-CNRS-BMBI). He explains the different models of artificial intelligence (AI).
Among the most widely used AI models are deep neural networks. “These models have the advantage of establishing a precise relationship between input and output data. However, the learning phase requires a large volume of data (measurements, observations, simulation results, contextual data…) and demands significant computing resources. This type of AI can be used to better understand the functioning of complex experimental devices where several physics are involved. Neural networks are capable of reproducing the general behaviour of multi-physics systems and enable sensitivity and optimization studies to be carried out on operating parameters”, he explains. In collaboration with Timothée Baudequin, the laboratory is planning to use deep neural networks to predict the behaviour of electrospinning devices. “These are machines that produce nanofibres to make scaffolds for cell cultures”, he says.
Other AI models used at UTC-CNRS-BMBI also include statistical learning or machine learning. “Members of the Characterization and Personalized Modelling of the Musculoskeletal System (C2MUST) team use this type of model to determine the behaviour of musculoskeletal systems and, for example, predict aging. It’s a probabilistic approach that takes account of uncertainties in models, data or experimental conditions”, stresses Florian De Vuyst.
Other AI tools are dedicated to assisting image processing, enabling the detection of anomalies or the search for important features in images, not necessarily visible to the human eye. “These can be static images or videos, but also dynamic 3D or even 4D images. This is one of Isabelle Claude’s areas of research, as she supervised Abdelhadi Essamlali’s thesis on bile duct reconstruction. It’s a patient-specific approach, where the patient’s organ is reconstructed in volume to help the practitioner prepare for surgery,” he adds.
It is often the application that determines the development of a particular type of AI. “Take, for instance the case of determining the mechanical behaviour of living tissue. Usually, we define one or more laws and try to find the one that best reproduces experimental measurements. Now, we use AI techniques in which we integrate these empirical laws into a family of more general laws, and the neural network finds the sub-family and coefficients that are closest to reality. In a way, it’s a general integrated approach that enables us to be more generalist and more precise when modelling a biological tissue”, he explains.
Finally and more recently, AI has been used to accelerate numerical simulations of mechanical models. “These include, for example, physics informed neural networks, which have the advantage of being more general and faster than conventional solvers such as finite element methods. In the learning phase of neural networks, a so-called loss function is used. In a PINN, the loss function is an equation residue. The problem is solved when the loss reaches zero,” he explains.
Today, AI models are used in most fields. Nevertheless, a certain amount of vigilance is still required with regard to possible biases. “AI should be seen as an assistant which, particularly in the biomedical field, can provide a complementary analysis or diagnosis that must imperatively be validated by human practitioners”, concludes Florian De Vuyst.
MSD