52: Machine Learning at the UTC-Heudiasyc lab
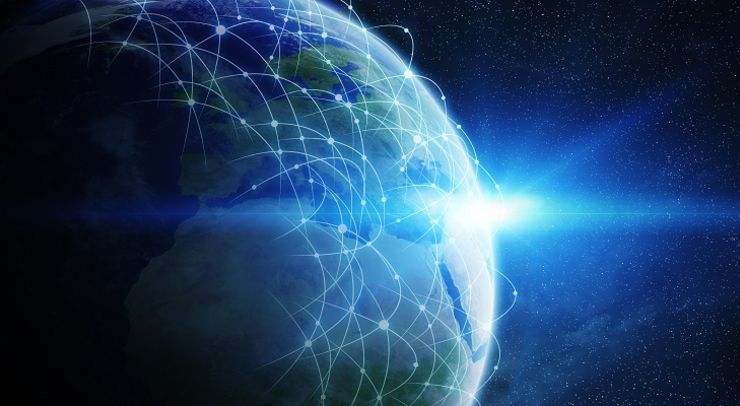
Professor Philippe Bonnifait, also Vice-Chairman of the scientific council of the University of Technology of Compiègne (UTC) former Director of a CNRS research group (GDR) in robotics between 2013 and 2017. Since January 2018, he has been Director of the UTC-Heudiasyc Laboratory, created in 1981. This state-of-the-art laboratory houses, in particular, the CID (Knowledge, Uncertainties, Data) team dedicated to research in artificial intelligence.

“Heudiasyc’s scientific scope has not changed much since its creation, but the themes have evolved. We focus on computer sciences in the broadest sense, with two main branches: the training of computer engineers and research Master students,” explains Philippe Bonnifait. This specificity explains the very strong link between the lab and the UTC’s computer science department, since the majority of the lecturers involved in the training of computer engineers (but also in the framework of the research Master’s degrees) report to UTC-Heudiasyc.
UTC-Heudiasyc is a cutting-edge laboratory that has seen “five of its research scientists seconded to the private sectors. Among them, two are at FAIR (Facebook AI Research), the firm’s laboratory in Paris,” he says. A laboratory whose lecturer-cum research scientists, with proven skills, provide quality training that is highly appreciated by students. The proof? “There are more than 700 matriculated computer engineering students. This is not insignificant. It is a highly valued generalist training that allows our students to easily adapt to the very rapid evolution of technologies in the sector. For Master’s degree students, the training is more specialized, closer to what we do in research, in short. Currently, Heudiasyc has 55 PhD students, which proves the interest, in the eyes of the students, in the research themes tackled by the laboratory,” he emphasises.
What are the major lines of research undertaken at UTC-Heudiasyc? “There are four priority themes: computer science, artificial intelligence (AI), a key word that has been around for 20 years, when it was not yet fashionable, and finally automation and robotics. All our teacher-researchers work in these disciplines,” says Philippe Bonnifait.
Since the restructuring of the lab in January 2018, three teams have been working on these themes: the CID team (Knowledge, Uncertainties, Data), the SCOP team (Safety, Communication, Optimization) and, lastly, the SyRI team (Robotic Systems in Interaction). “The first team is dedicated to what we do in the foundation artificial intelligence studies, understanding that not all areas of artificial intelligence are addressed. We can mention machine learning, interactive learning, recommendation systems, etc.” The second works in particular on the problems of scheduling, networks but also — and this is a novelty in the laboratory — on safe systems, in other words, systems that are failsafe and secure. As systems communicate increasingly, data exchanges are therefore important. “Hence the major challenge of securing them against an attack by hackers, for example. Finally, the last theme addresses those questions that revolve around the autonomy of machines, in particular the articulation between robotics and artificial intelligence, the first being in the physical world while the second, computational, virtual, is located in the “cloud” or in computers. We now speak of artificial intelligence embodied by robots,” he explains.
The choice made by Heudiasyc in the vast field of artificial intelligence applied to robotics? “We chose to focus our research on mobile robotics, particularly those dedicated to transport and mobility. Today, we are talking about intelligent (“smart”) and autonomous vehicles. Vehicles designed to drive in shared environments. We were also one of the first labs to launch, in 1997, into UAVs,” he explains.
A choice that allowed the lab to participate, as part of the Government Incentive Programme Investments for the Future (PIA), via Labex MS2T and EQUIPEX to Robotex and enabled Philippe Bonnifait to pilot everything related to land and air mobile robotics in France. A project launched in 2011 and running until the end of 2021 which also had the support of the Hauts-de-France Region to the tune of 3.5 million euros in scientific equipment.
This policy choice that naturally led to a large number of industrial partnerships, particularly in the field of transport. “With Renault, for example, within the framework of Sivalab, a joint Renault/UTC/CNRS laboratory, or a ten-year project, launched in September 2019, with the IRT Railenium consortium related to the concept of autonomous trains,” concludes Philippe Bonnifait.

As a graduate engineer from École des Ponts ParisTech (ENSP), Thierry Denoeux pursued a PhD thesis on “the reliability of rain forecasts by weather radar” in a laboratory dedicated to the environment at ENPC. He is interested, among other things, in computer science, pattern recognition and image processing. “The goal was to process radar images to analyse and extrapolate the movement of rain cells for quantitative forecasting in the very short term (one to two hours). These predictions were used to optimize the management of large sewer systems in order to limit flooding in the event of a storm,” he explains.
An interest that naturally led him, after his PhD, to join the Laboratoire d’Informatique Avancée de Compiègne (LIAC), Lyonnaise des Eaux, which has since become Suez, as a research engineer. He stayed there for three years and worked on European projects with lecturer cum-research scientists working at UTC, in the early 1990s, when artificial intelligence (AI) was already arousing a lot of interest with the development of expert systems.
He joined UTC Compiegne in 1992 as a contract lecturer-cum-research singulier scientist at Heudiasyc before being appointed as full professor in 1999. Several responsibilities followed suit: director of a joint laboratory with Suez, deputy director of Heudiasyc, vice-president of the scientific council of the UTC, scientific coordinator, before taking over as director in January 2019, of the Labex Maîtrise des Systèmes de Systèmes Technologiques (MS2T) — a tenyear project — which, as part of the Government Incentive Programme Investments for the Future (PIA), running until 2021. Also in January 2019, he took over the management of the SHIC¹ research federation, a CNRS structure initially grouping together the mixed Heudiasyc, BMBI and Roberval units, which were recently joined by the Costech unit. This federation provided impetus to a new dynamic for interdisciplinary technological research within the UTC.
At UTC-Heudiasyc, Thierry Denoeux is part of the CID team in charge of artificial intelligence, structured around two main research areas. The first concerns knowledge and data processing with themes such as knowledge modelling, machine learning and uncertainty management, a major challenge in both artificial intelligence and statistics. “Indeed, how can we model uncertainty, reason and make decisions knowing that we do not dispose of all the information needed ?” he says. The second area of research is concerned with customized adaptive systems. In other words, everything that relates to the interaction between humans and systems with the idea of designing systems that can automatically and dynamically adapt to the user and the context of use.
Thierry Denoeux works mainly on the first priority theme. “I work essentially on the modelling of uncertainties in intelligent systems, a theme that lies at the interface between artificial intelligence and statistics. I am particularly interested in the theory of belief functions, a theory of uncertainty that allows us to reason and make decisions in the presence of uncertainties. It is a general theory, which encompasses probability theory, and has many applications because uncertainties are ubiquitous. Research in this area is multidisciplinary and involves economists, AI specialists, statisticians and others,” he explains.
A field that led him, in 2010, to participate in the creation of a learned society Belief functions and Applications society (BFAS), an association of which he is the President. The objective? In particular, to promote teaching, research, the advancement of knowledge in the field of belief functions and to explore the links with other theories of uncertainty. Hence the launch of international conferences held every two years — the next one will be convened in Shanghai in 2020 — and a thematic school for the training of PhD students, the latest edition of which was held in October 2019 in Siena (Italy).
However, Thierry Denoeux does not confine himself to the theoretical aspect of his research on belief functions, as he is also interested in the concrete applications that can derive from them. One example is automated postal address recognition, which was the subject of a CIFRE thesis in partnership with Solystic, one of the world leaders in the provision of automated sorting and distribution preparation solutions for parcels and mail. “This company sells machines with handwritten address recognition software. So when the address is not recognized, the envelope is rejected and processed manually. The challenge is to reject as few envelopes as possible while making as few errors as possible on those that are accepted. To meet these two criteria and improve machine performance, the idea was to integrate several software programs and combine the results of these systems using belief function theory,” he explains.
Other applications include the work carried out with the French Institute of Transport, Planning and Network Science and Technology (IFSTTAR) and the SNCF on “Diagnosis of railway track circuits”, and the ongoing collaboration with the Laboratory of Computer Science, Information Processing and Systems (LITIS) of the University of Rouen on “Segmentation of tumours in medical images and prognosis based on the evolution of patient data”. The theoretical corpus of belief functions is of course also of interest to the SyRI (Robotic Systems in Interaction) team, which is working in particular on intelligent vehicles (IV). “One of the problems in IV concerns perception. IVs are full of sensors and the challenge is to be able to process the information collected by these sensors to recognize objects on the road such as pedestrians, cyclists, etc. One of the problems in IVs is perception. We therefore need to combine the information from these different sensors. And here again, there is a lot of uncertainty, because each sensor provides partial and sometimes unreliable information about the environment,” adds Thierry Denoeux. Should we be afraid of AI? “Irrational fear is irrelevant. However, some applications of AI pose ethical problems, such as generalised video surveillance with, in particular, the development of facial recognition. As biologists have been doing for a long time, computer scientists must now be concerned about the ethical implications of their work,” he concludes.

A field that she has been working on since her PhD thesis at the École des Mines de Paris: a CIFRE thesis funded by the SNCF on “Virtual Reality and Training of TGV Drivers”, conducted between 1998 and 2001. She pays particular attention to the pedagogical objectives or “how,” she says, “virtual reality could either improve existing training or complement it”.
Initially trained as a computer scientist, she immediately refused to address this question solely from a technical point of view. “I started working with ergonomists, education specialists and also end-users,” she explains. Once her PhD was completed, she stayed for four years at the École des Mines as an associate research officer, joined UTC-Compiegne in 2005 as a lecturer and continued her research based on concrete needs, always in conjunction with industrialists.
Her first project resulted from a meeting with researchers from the National Institute for Industrial Environment and Risks (Ineris). The objective? “To ensure the training of subcontracted operators who work on high-risk sites. This was all the more topical because of the explosion at the AZF plant in Toulouse, a tragedy that happened only a short time earlier. Explosion due to human errors in subcontracting,” says Domitile Lourdeaux. A project that mobilized three PhD theses and obtained significant funding from the National Research Agency (ANR) but also from the Region. “Usually, virtual reality is used to train in a technical gesture or procedure. But I wanted to distance myself from this schema. Since we are in high-risk areas, I wanted the learner to be able to make, possibly make mistakes and see the consequences of his mistakes,” she adds.
Since then, Domitile Lourdeaux has been working on a series of new projects. This is illustrated by one on “training aeronautical operators in aircraft assembly” in partnership with STELIA Aerospace (Méaulte). Another innovative project? VICTEAMS (2014–2019) conducted in partnership with LIMSI in Orsay, specialists in cognitive ergonomics, the Val-de-Grâce military medical school and the Paris City Fire Brigade. Project that will surely be pursued.
What is the particularity of the STELIA and VICTEAMS projects? The answer is the level of integration of artificial intelligence (AI). “Since we couldn’t afford to put dozens of developers, like video game manufacturers for example, we came up with the idea of integrating AI to create scenarios,” she points out. “Prior to the STELIA project, the trainer selected the level of the learner and then gave him/her the learning objectives, for example, to work on a particular safety rule. The scripting system then generated learning situations based on these objectives. In the aeronautics project, we wanted to create a dynamic learner profile, i.e., to ensure that the system was able to detect the learner’s skills in order to propose interesting situations. We therefore start from beliefs about his ability to manage, or not, the situations he/she is confronted with. This is called the “proximal zone” of development. In other words, that one has skills and is capable of acquiring new skills, close to one’s own skills. To do this, genetic algorithms and belief functions have been used to gradually extend this proximal zone,” she explains. With VICTEAMS (see box), Domitile Lourdeaux goes further. “I wanted to emphasize collaborative work by creating virtual teams. The involvement of different players and their interaction requires an even finer degree of scripting. This is notably the case for training medical leaders in the management of a massive influx of injured people,” she explains. Read also on the same subject Machine Learning at the UTC-Heudiasyc lab Machine Learning at the UTC-Heudiasyc lab L’UTC forme les nouveaux talents de la cybersécurité THEME : :ICTS: COMPUTER SCIENCES; AUTOMATION & CONTROL; DECISION THEORY AND APPLICATIONS L’UTC forme les nouveaux talents de la cybersécurité VR immersion THEME : :ICTS: COMPUTER SCIENCES; AUTOMATION & CONTROL; DECISION THEORY AND APPLICATIONS VR immersion

After his DEA (currently the Master 2 diploma) in Artificial Intelligence (AI), Sylvain Lagrue has been working on a European project on “Taking uncertainties into account for preference modelling in geographic information systems”. After completing his thesis, he joined the University of Artois in 2004 as a lecturer before joining the UTC as a full professor.
His role within the CID team? “My crossdisciplinary profile allows me to work with the different researchers in the team. Both in the field of “the uncertain” and that of “knowledge representation”, for example,” he says.
And how does AI fit concretely into all this? “For the public at large, AI is magic made by the computer. And the more magical it is, the more AI. In other words, seeing actions made by computers that we thought were impossible,” he says.
One example among others? “Let’s consider games. When IBM’s Deep Blue defeated world chess champion Kasparov in 1997, the general public thought that AI was going to take everything in its path, and then it calmed down. The reason? It was noticed, after analysis, that what won in 1997 was the computer’s computing capacity. For the general public, this is no longer magic. So it’s no longer AI,” he explains.
But then what is AI in his mind? “It’s about making a machine reason when you don’t expect it to be able to do it. So there’s a whole aspect of logic, but also of decision making. In a word, making it reason and make intelligent choices,” he describes.
This is reflected in its three areas of research. The logical representation of knowledge and reasoning? “Logic has always — since ancient times — been a way to formalize reasoning based on a certain number of hypotheses allowing us to draw conclusions that are valid. Our objective is to see this type of advanced reasoning done by a machine. This can be achieved efficiently thanks to resolution and deduction algorithms which, based on the hypotheses, ultimately allow a machine to make decisions”, stresses Sylvain Lagrue. A skill that has led him to work on a European project aimed at “safeguarding intangible heritage in South-East Asia and in particular the Water Puppets of Vietnam, whose playlets represent the country’s history, legends, scenes from daily life, etc. “All this is accompanied by music, songs and recitations. In terms of richness, they can be compared to opera in Europe. So we had to represent a lot of complex knowledge,” he says. Managing uncertainty with AI? “If you roll a die, you don’t know which side it’s going to fall on. However, in this case, we do have probabilities. In other cases, we don’t even have probabilities. In the formalisms that I use, the challenge is to model a sequence of “we think that such and such an action leads to this but in the opposite case rather to that”. In short, a much more ordinal modelling,” he says. Finally, is there an interest in AI games? “The advantage of gaming? It allows us to have a controlled universe. You know what environment you’re in, with its precise rules, whose effects you know, and you don’t have to worry about the physical aspects. It allows us to test a large number of algorithms,” he explains. An interest that led him to co-direct a thesis on “general game playing”, or how to make a computer play any game. “Deep Blue could only play chess, for example. In order to develop a program capable of playing all games, we had to represent all games with complete information thanks to the Game Description Language (GDL). Which brings us back again to the representation of knowledge”, concludes Sylvain Lagrue.